Can a Biotechnologist Leap into Data Science Success?
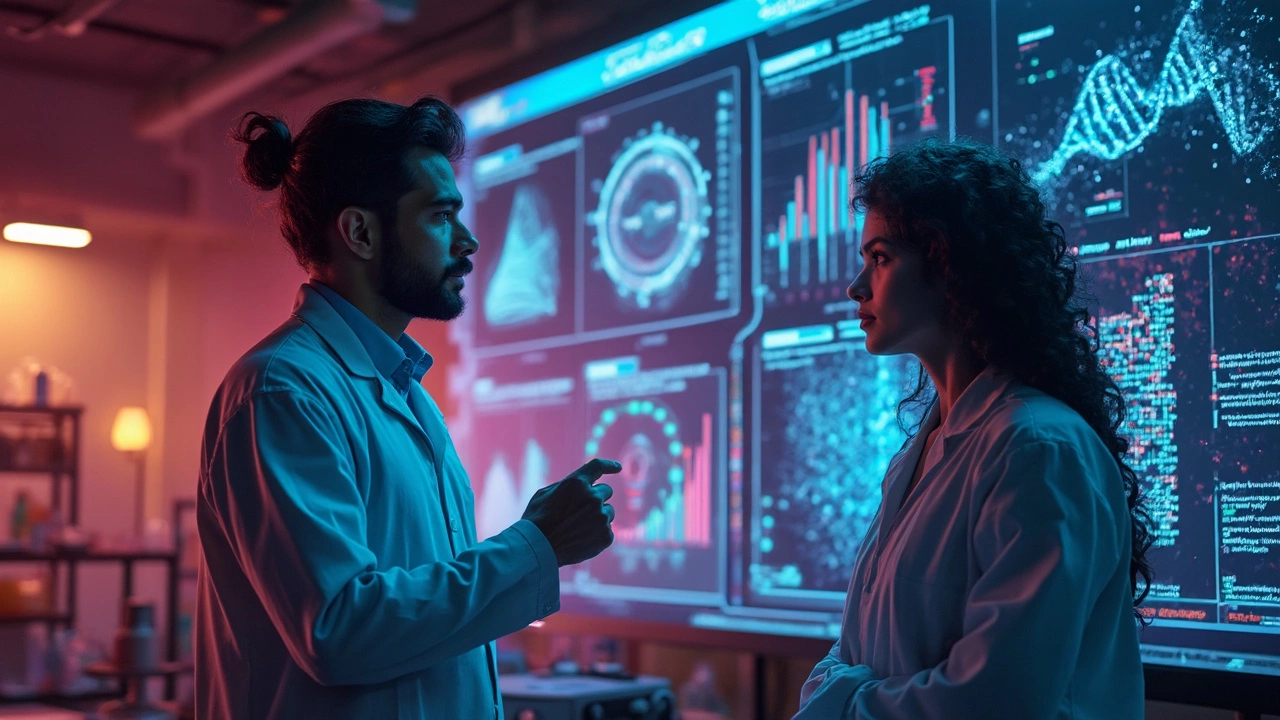
In our ever-evolving tech-driven world, changing careers isn't as daunting as it once was—especially for folks in biotechnology looking to step into data science. Just think about it: both fields love data almost as much as cat lovers adore their pets. If you're a biotechnologist, your daily routine already involves wading through mountains of data, drawing conclusions, and making decisions backed by evidence. Sounds familiar? That's where data science comes in, promising new puzzles to solve with your trusty analytical skills.
Now, before we get all hyped up about switching lab coats for coding, it's worth knowing that this transition isn't just about picking up a few Python courses. It's about learning how to think like a data scientist—seeing the world through numbers and probabilities. Don't worry, though. Being a biotechnologist means you're already curious and investigative by nature, so you're halfway there!
- Biotech Basics and Data Science
- The Skill Overlap
- Re-training and Education
- Navigating Career Challenges
- Success Stories and Tips
Biotech Basics and Data Science
Alright, let's kick things off by understanding what these two worlds are all about. Biotechnology is all about using living systems and organisms to create or modify products. Think of it as nature and science teaming up to make awesome stuff like better medicines, pest-resistant crops, or even biofuels. It's a field deeply rooted in research, data collection, and innovation.
Now, slide into the world of data science, and you'll see it's all about extracting insights from data using techniques like statistics, machine learning, and predictive modeling. Data scientists don't just stare at numbers; they make sense of them and help businesses make smarter decisions.
Both biotechnologists and data scientists love diving into complex data to solve problems. The skill sets often overlap, especially when you're dealing with bioinformatics, which blends biology, computer science, and information technology. Bioinformatics is how researchers decode genomes, which means they need to know a bit about data science to manage those enormous datasets.
Fun fact: Biologists and data scientists often work together. How else would someone turn genome sequences into life-saving treatments, right? Imagine analyzing billions of DNA sequences. That’s a lot of data, and it requires skills that both biotechnologists and data scientists excel at: keen analytical skills, an eye for detail, and creative problem-solving.
This unique overlap means a biotechnologist with a knack for data might look at a data scientist role and find it surprisingly familiar. It's kind of like when Whiskers, my dog, spots a squirrel in the park—there's a mix of curiosity and excitement about what comes next.
The Skill Overlap
Alright, let's talk about where the worlds of biotechnologist and data scientist meet, which is more often than you'd think. If you're knee-deep in bio-data and genomic sequences as a biotechnologist, you've already got a leg up in handling complex datasets. Biotechnology is all about understanding life processes, and to do that, analyzing data is key. This means you already know how to play around with numbers and make sense of them, much like data scientists do.
Biotechnologists often work with statistical analysis and experimental design—core components in data science. Being able to dissect experimental results and spot patterns is no small feat; it's the bread and butter of data science too. Plus, biotech folks tend to have a solid foundation in mathematics, which is crucial when you're diving into machine learning algorithms and predictive models in data science.
Let's not forget project management and collaboration skills. Biotechnologists regularly collaborate across different fields, from clinical labs to research facilities, which is very similar to how data scientists work in cross-disciplinary teams with engineers, analysts, and business stakeholders.
- An understanding of data ethics is another overlap. Whether you're managing patient data in biotechnology or consumer data in data science, respecting privacy and ethical guidelines is a shared responsibility.
- A knack for problem-solving connects both fields. Whether forming hypotheses in a lab or predicting customer behavior, the mindset remains the same.
Now, if you're wondering about practical skills—coding might be the new kid on the block. But don't fret; if you've tinkered around with R or any bioinformatics tools, you've got a great start. These tools introduce you to the necessary logic, making the coding transition smoother.
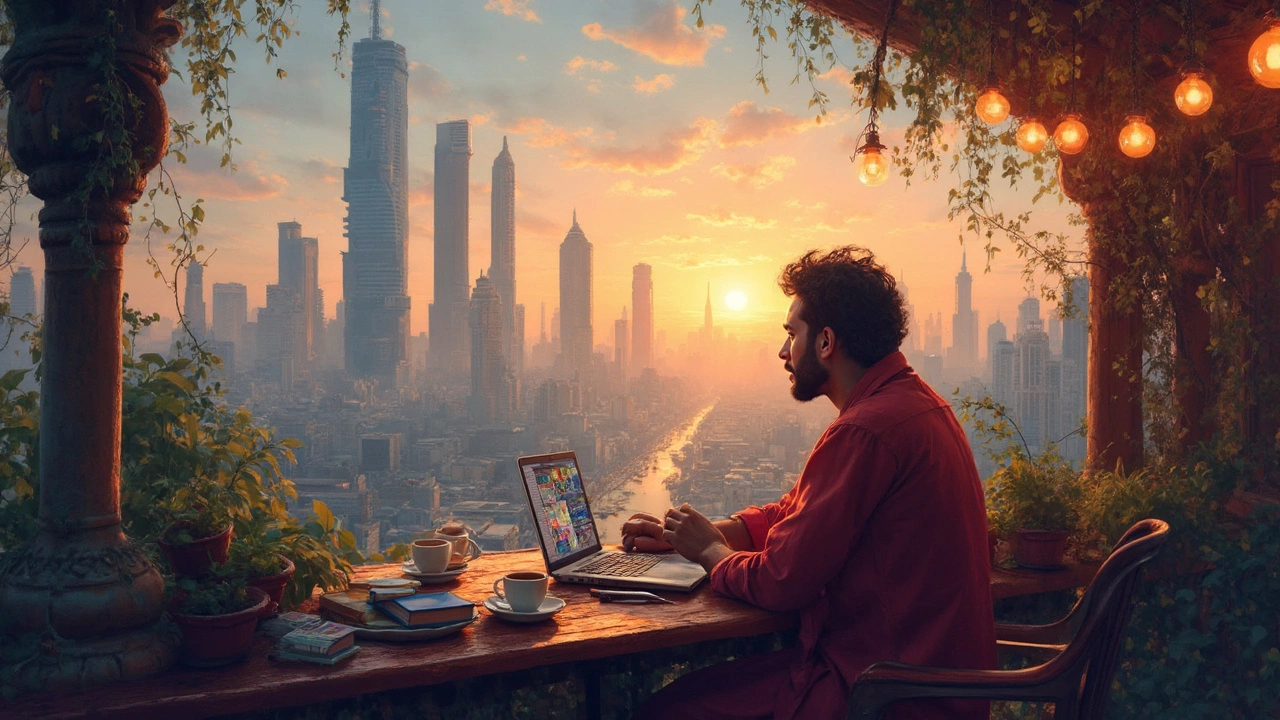
Re-training and Education
If you’re a biotechnologist toying with the idea of becoming a data scientist, don’t worry about starting from scratch. Most of your base skills are already there. However, brushing up on some tech-savvy skills is key. You’ll want to get comfy with programming languages like Python and R. These languages are like the bread and butter of data science. Lucky for you, there's a mountain of free online resources and courses you can devour at your own pace.
Consider enrolling in a data science bootcamp. With the number of these growing each year, you can find one that suits both your schedule and budget. Bootcamps are immersive and shorter than traditional degrees, focusing intensely on the practical aspects of data science. This can be a game-changer if you're looking to switch fields fast.
Andrew Ng, co-founder of Google Brain, once said, "Data science is a discipline that requires skill building, but is incredibly rewarding and impactful."
Don’t forget the importance of hands-on practice. Joining platforms like Kaggle for competitions can make a world of difference. By diving into real-world datasets, you get to flex those coding muscles and think like a data scientist. It might feel a bit like being thrown into the deep end at first, but that’s often the best way to learn.
Check out online communities and forums too. Rubbing shoulders with other data enthusiasts can be a great way to learn new tricks of the trade and stay ahead in this ever-evolving field. When others share their experiences and projects, it sparks ideas and at times, helps solve a problem you might be stuck on.
Lastly, don’t underestimate the power of a good book. Texts like "Python for Data Analysis" by Wes McKinney can be a goldmine of knowledge for anyone looking to dive deeper into the world of data. Adding these to your reading list can supplement everything you’re learning.
To wrap up, while transitioning from biotechnology to data science requires some effort, it’s definitely not an Everest climb. With the plethora of resources available today, combined with your existing analytical skills, you’re not only making a feasible leap but an exciting one!
Navigating Career Challenges
Making the jump from being a biotechnologist to a data scientist sounds exciting, but let's be real—it comes with its share of bumps and hiccups. For starters, adjusting to a new mindset can be tricky. You might be used to wet labs and bench work, but now you'll need to embrace the keyboard more than the pipette. But, hey, change is good, right?
One of the biggest hurdles is getting comfy with programming languages, especially ones like Python or R, which are the bread and butter of data science. Don’t fret—there are tons of resources to help you out, like online courses and coding bootcamps tailored for folks in the biotech and science domain. Trust me, once you get the hang of loops and functions, it becomes pretty addictive.
Besides the tech skills, understanding the business or practical implications of data is crucial. It's not just about running the numbers but making sense of them in a way that helps companies make decisions. This means learning how to tell a compelling story with data—think of it like presenting your biotech research, but with graphs instead of gels.
- Find mentors or peers who've made the switch. They can offer real-world insights and support.
- Build a portfolio. Start with small projects or datasets related to your current field to showcase your analytical chops.
- Network with data science communities—LinkedIn groups or local meetups are great for connections and advice.
Oh, and let's talk about expectations. It might feel like starting from scratch, but the key is to leverage your biotech background. Highlight how those hours in the lab honed your research skills and attention to detail, which are gold in any data science job.
If you’re still not convinced, consider this: many backgrounds combined have shown they’re more innovative because they bring different perspectives to problem-solving. Who wouldn't want to offer something unique in the fast-paced world of data? Keep at it, and remember, every expert was once a beginner.
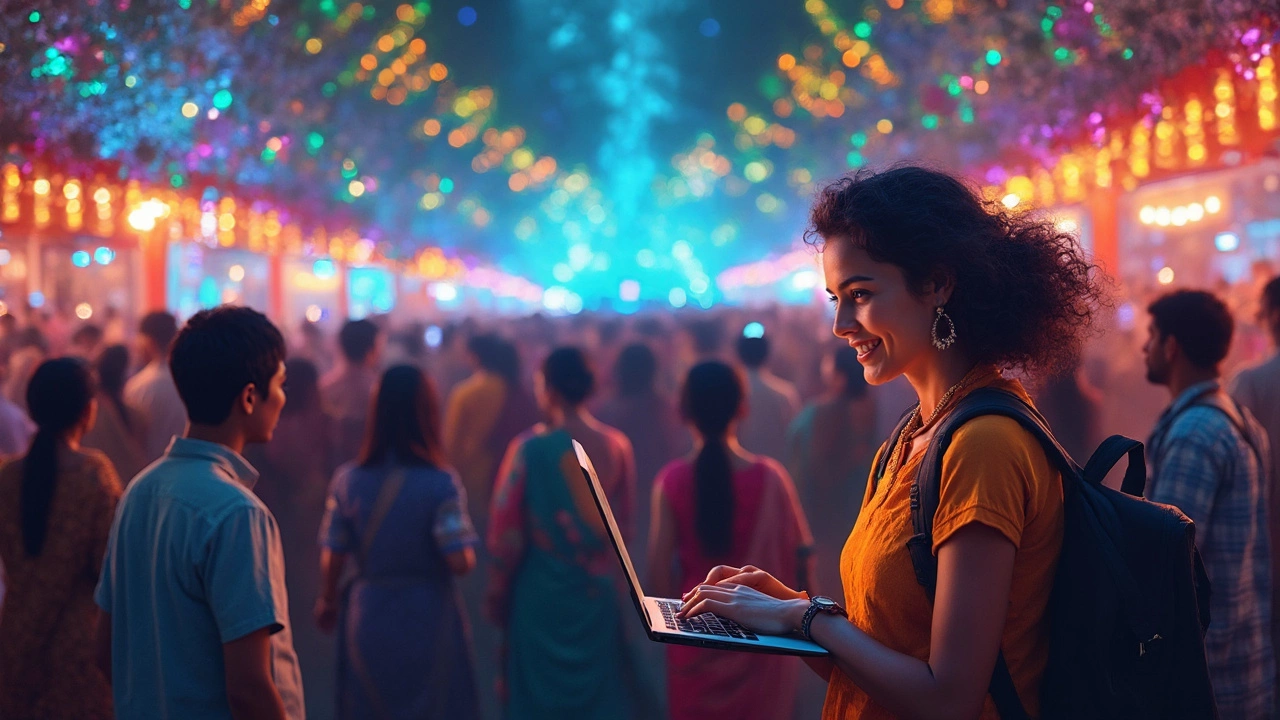
Success Stories and Tips
Let's kick things off with some inspiration from real-life biotechnologists who've made this leap. Take Emma Hughes, for instance. She switched from studying genetic markers to finding insights in healthcare data. Her strong foundation in data analysis back in the biotech field gave her a head start in data science. She focused on gaining new tech skills through online courses in Python and machine learning, which turned out to be incredibly valuable.
Then there's Raj Patel, who transitioned into predicting agricultural yields through data science. His biotechnologist background in plant genetics made him exceptional at understanding complex datasets. He advises budding data scientists to capitalize on familiar skills like critical thinking and problem-solving while learning to code and understand algorithms.
So, how do you make the transition? Here are some tips:
- Build on Your Existing Skills: Your ability to interpret data and conduct experiments in biotech will be useful in data scientist roles. Focus on statistics, pattern recognition, and hypothesis testing.
- Learn New Tools: Get comfy with tools and languages like R, Python, SQL, and Tableau. They're often the bread and butter of a data scientist.
- Consider Formal Education: Bootcamp courses or certifications in data science can provide structured learning and connections within the industry.
- Network and Mentor Up: Engage with communities, attend meetups, and find mentors who have walked this path. Their guidance can be priceless.
Data scientist roles often require a mix of new skills and your already-honed abilities. While it's no walk in the park, many find it worth the effort, combining the logic of biotech with the tech-savvy world of data. Whether through online classes or real-world projects, biotechnologists can successfully navigate this new career landscape.